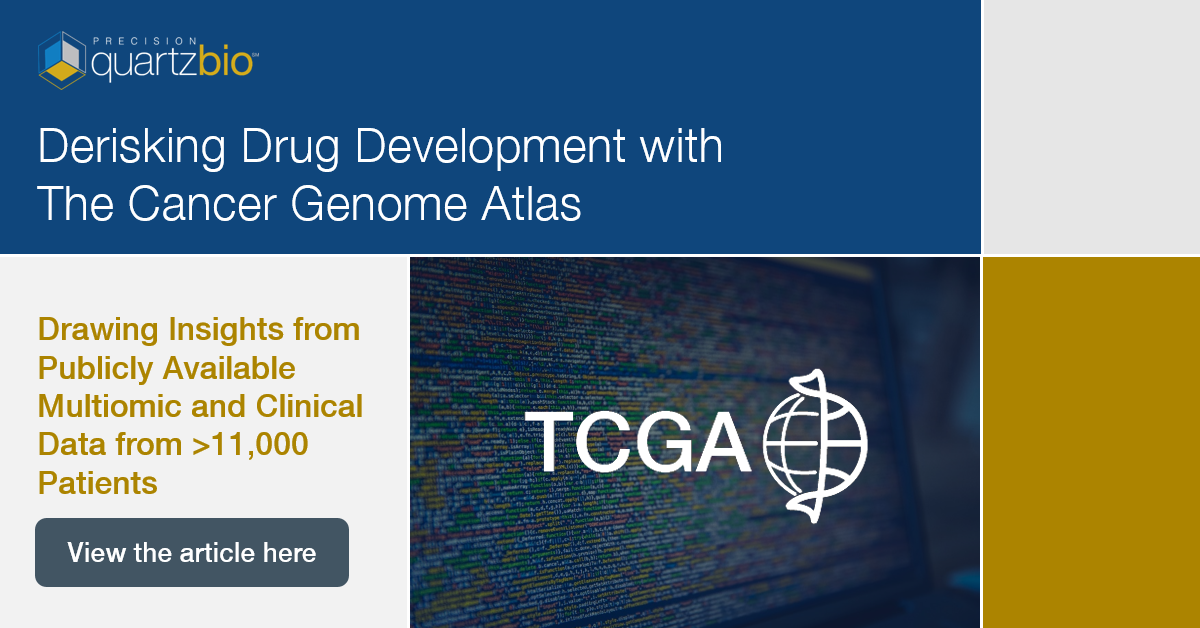
January 27, 2021 — Non-profit and governmental organizations have invested immense financial and labor resources, with generous contributions from participants, to create large-scale data sets. The Cancer Genome Atlas (TCGA) is one such publicly accessible resource, and represents a uniquely comprehensive collection of multiomic and clinical data, from which drug development researchers can derive knowledge about disease, treatment, and the biology of individual tumors.
Enabling Insights with Publicly Available Multiomic and Clinical Data from >11,000 Patients
At this time, the data set was derived from sample contributions from over 11,000 cancer patients, representing 33 primary tumor types. In many cases, analogous measurements have been generated from tumor-adjacent tissue samples, which is an invaluable comparator not frequently found in other data sets. In total, TCGA has over 2.5 petabytes of molecular data, comprised of genomics, transcriptomics, epigenomics, and proteomics; coupled with rich clinical annotations and metadata, including demographics, treatment and exposure history, survival data, and biospecimen records.
Figure 1. The Cancer Genome Atlas
![]() |
>11,000 cancer patients providing 33 primary tumor types & matched normal tissue samples |
>2.5 petabytes of ‘omics data – with genomic, transcriptomic, epigenomic & proteomic data |
Clinical metadata including: demographics, treatment & exposure history, survival data, and biospecimen records |
---|
The challenges of working with a data asset of this level of molecular and clinical complexity include
- Data Management – cleaning, extraction and integration.
- Data Processing – quality control, mapping, and normalizing across multiple study types and platforms.
- Knowledge – application of previous scientific insights to the data set, or generation & recording of new insight from the data set.
- Computation – the application of mathematical approaches to generate reasonable, testable hypotheses from all of the data; both correlative analyses (e.g., machine learning, correlative network reconstruction) and knowledge-driven causal analyses (e.g., Bayesian approaches, reverse causal inferencing).
- Biological Interpretation – this requires knowledge of biology in order to investigate the veracity and likelihood of truth behind a hypothesis generated from a computational model.
- Application – direct implementation of these insights to a challenge or hurdle within the organization (e.g., selection of the animal model that best recapitulates clinical pancreatic cancer tumor biology).
Accordingly, a deep understanding of the strengths and limitations of this extraordinary asset is crucial to efficiently and accurately build and interrogate the appropriate analysis data set to draw meaningful insights from such a complex resource in order to advance development goals. This requires multi-disciplinary expertise, a technically robust infrastructure in order to execute in the areas listed above, as well as time spent tracking down, reading about, and implementing computational approaches with the different versions of data that have been released over the years.
We have tackled these challenges by building a technology-enabled platform as follows:
Figure 2. Three Key Components to Draw Actionable Insights from Public Data Assets
![]() Data Integration Engine to build analysis data sets |
![]() Proprietary Knowledge Engine to build analysis data sets |
![]()
Biological Expertise to prioritize insights by therapeutic relevance |
---|---|---|
The QuartzBio team deploys technology-enabled pipelines that integrate survival outcomes and clinical annotations, map data between modalities, and integrate TCGA data with other public and proprietary data sources, including:
|
QuartzBio leverages decades of scientific knowledge and research dollars through a computable knowledge graph of cause-and-effect biological relationships:
|
Algorithms themselves are an important part of computational biology – but the intensive legwork comes from:
The latter requires years of training in molecular biology, not just systems biology. |
Although TCGA data set may be difficult to work with for newcomers, it remains the pre-eminent public resource that oncology researchers should consider leveraging to deliver actionable R&D intelligence. Examples of how we have worked with clinical and translational teams are outlined in the figure below.
Figure 3. Examples of Derisking Programs through Translational Intelligence with TCGA
Patient Cohort Selection & Immunobiology |
|
Indication Matching & Line Expansion |
|
Biological Modeling of MoA & Competitive Differentiation |
|
Prioritize Translational Model Systems |
|
TCGA is a powerful resource for enabling pre-clinical, translational, and clinical teams to interrogate their own data against an independent data set, or across therapeutic areas using a resource that would have been nearly impossible to budget for exclusively within their organization – both in cost and time.
Our team views public data resources as incredible assets that are maximized when advanced integration and mapping pipelines are combined with a knowledge engine that also enables the research team to pull in other public or private sources of data. In our next blog post, we will describe specific technologies we have built to rapidly deliver translational insights that might be missed using traditional TCGA analyses.
We are curious to hear how you think about leveraging public data sets. What has your experience been like? Are there applications or obstacles that have not been addressed here?