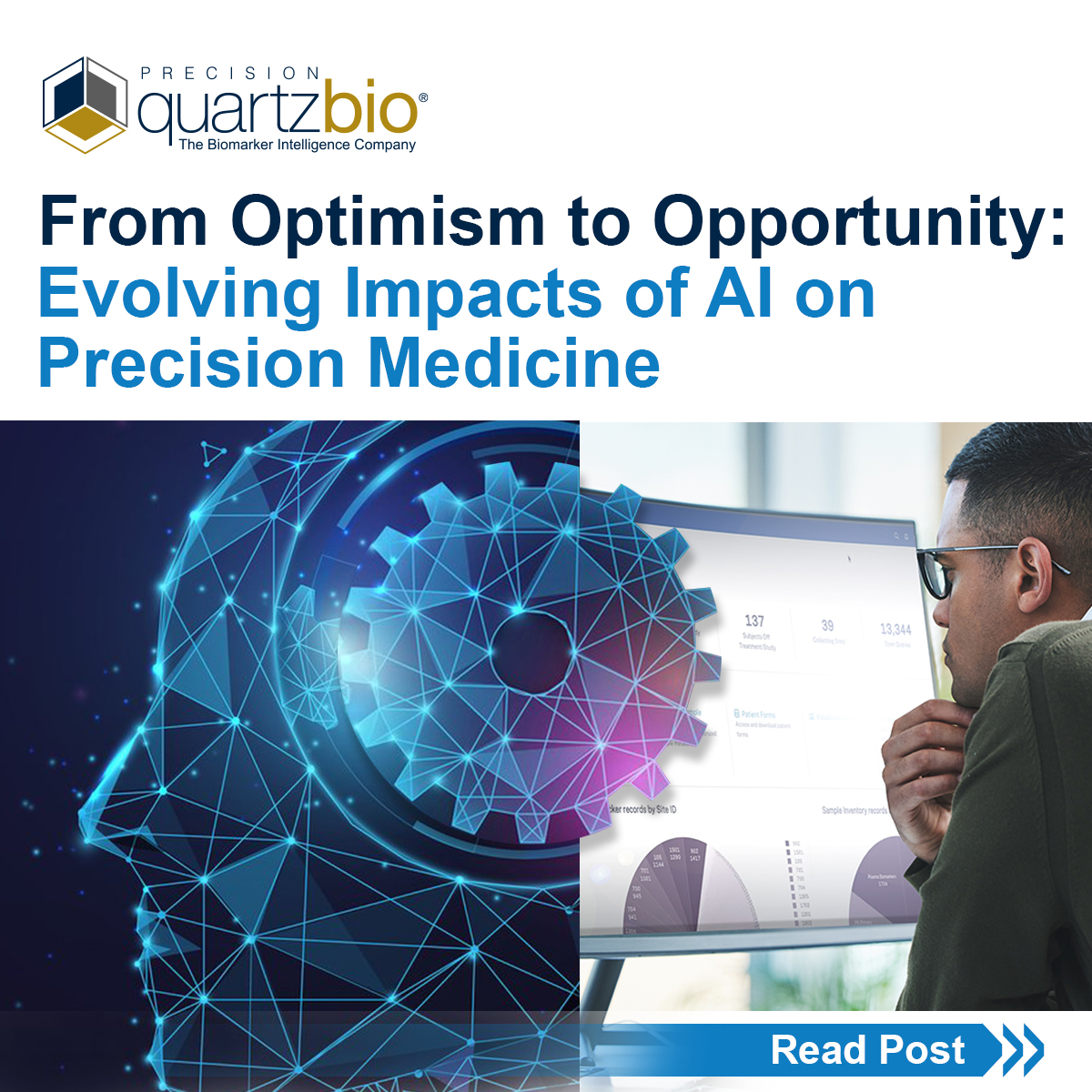
It was late in the afternoon, a windy day in Boston, as we listened to precision medicine development leaders within pharma and biotech spaces get excited about applying AI to their day-to-day work.
“We’ve gone from optimism to real opportunity here,” said one precision oncology team lead, eliciting nods across the room.
The explosion of generative AI and, specifically, large language models (LLMs), has created renewed energy, focus and promise around the hype of AI impacting the R&D lifecycle. Generative AI is revolutionizing precision medicine clinical trial planning, execution, and operations. Organizations are increasingly recognizing its potential, rallying behind AI initiatives. The race is on – the race to leverage AI as a force multiplier, knowledge amplifier and value generator, ultimately accelerating time-to-insight.
How can generative AI deliver on these promises?
Applications of generative AI and LLMs in precision medicine
AI-enabled technologies are empowering precision medicine development teams to make better decisions, faster, thanks to AI augmentation of human efforts in data processing, pattern recognition, insight generation, broadening of use cases, and performing complex queries.
As a result of these capabilities, the list of use cases for generative AI in drug development grows longer each day: drug target identification, target prioritization, patient selection, trial design, protocol generation, site management, trial monitoring, biomarker data analysis, regulatory submissions, and more.
Example use case 1:
Extracting insights from previous precision medicine clinical trials to inform subsequent studies. AI and machine learning algorithms can help researchers organize and interrogate billions of existing clinical trial data points, including clinical annotations of collected samples, information from scientific literature, and exploratory biomarker data, before a new clinical trial begins.
The insights from these data sets can help identify drug targets, define the patient populations most/least likely to respond, and even identify relevant, stored clinical samples from closed studies that have been consented for future biological research.
Example use case 2:
Optimizing operational efficiency of precision medicine clinical trials. Biomarker-informed clinical programs depend on the right samples and their associated data / metadata trails arriving at the right place, at the right time.
Augmenting the hard work of human clinical operations, biomarker and sample operations teams, AI-enabled technologies can monitor sample collection, informed consent and compliance, data generation, and data quality, surfacing inconsistencies in time for intervention.
Human power, optimally utilized via efficient workflows and streamlined processes, remains a key determinant of the success of a clinical program. Our goal is to establish a framework in which AI-enabled technologies amplify the talent and knowledge of human teams.
QuartzBio’s approach: amplifying talent and knowledge with generative AI and LLMs
As domain-specific LLMs proliferate, precision medicine development organizations are discovering that these technologies have the most impact when they enable not just one team, but key stakeholders across the entire precision medicine R&D lifecycle – human-centricity and AI as an amplifier is a key focus of many organizations. In the words of Samer Ansari, Takeda Oncology’s Head of Data, digital transformation is “really about elevating the human experience.”
Organizations are moving away from point solutions, each of which address a narrow use case, and towards platform-based solutions with two key characteristics:
- Platform-based solutions address broad use cases, such as the entire precision medicine development lifecycle.
- They can be easily deployed in the space of a more broadly connected, interoperable data and technology ecosystem.
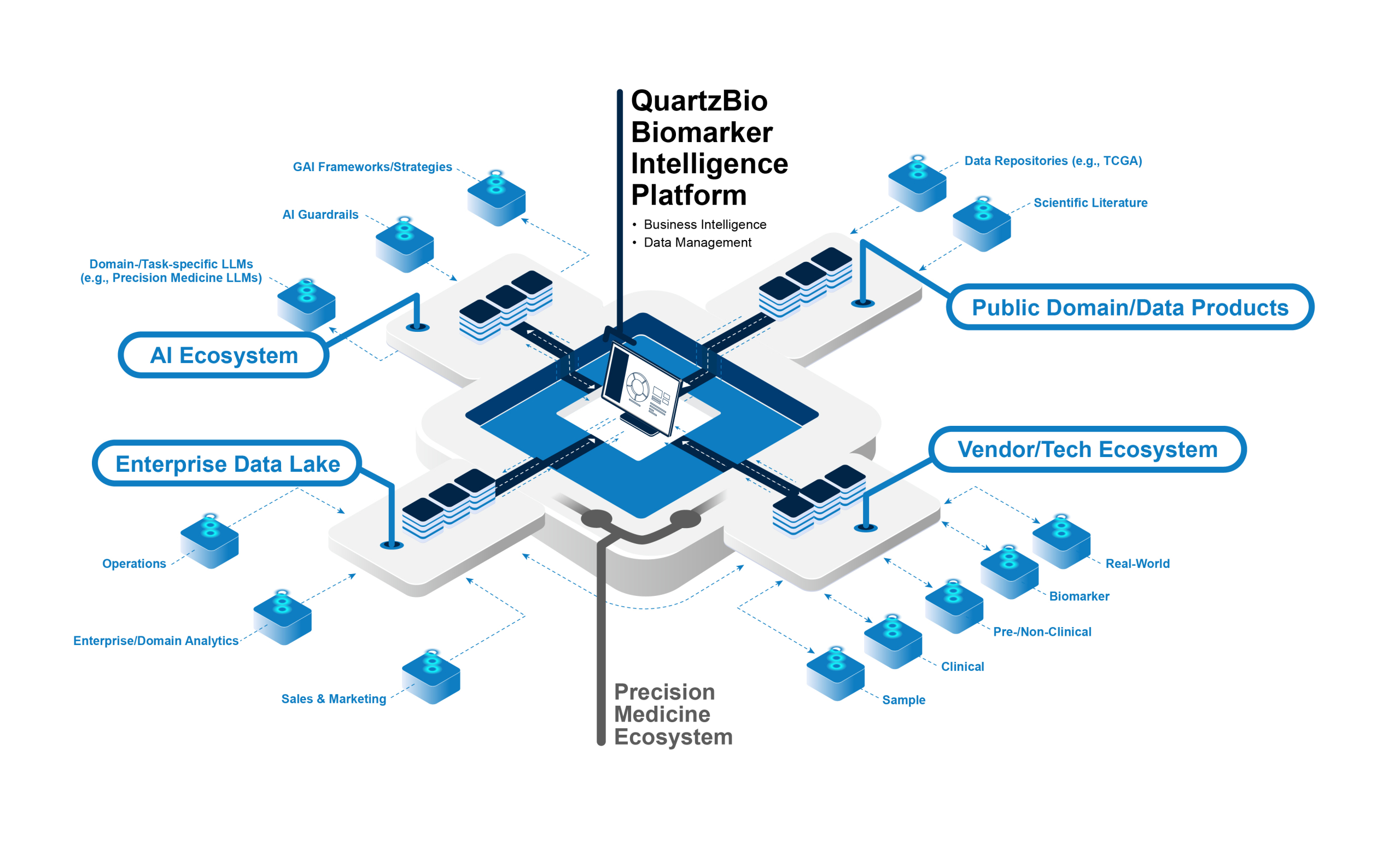
The industry’s experience with traditional technology applications supports the need for a unifying AI-enabling framework. The average organization uses 130 different software applications, and the average worker must toggle between applications over 1,200 times a day. The result is that information and insights stay in siloes, and teams exert enormous effort just to connect data and technology.
Imagine an AI-enabled precision medicine intelligence platform, optimized for augmenting the daily work of biomarker operations teams, translational scientists, sample operations teams, data scientists, R&D IT teams, and executive-level stakeholders.
Such a tailored platform would multiply the force of each team member by empowering them with role-specific insights, based on high-quality, interconnected data, while also making it as easy as possible for teams to work together. This is the vision behind QuartzBio’s next-generation approach to generative AI for precision medicine intelligence.
Three pillars of precision medicine intelligence: conversational, prescriptive, and navigational AI
There are three core concepts that form the foundation of our approach to precision medicine intelligence: conversational, prescriptive and navigational AI.
- Conversational AI enables users to conversationally interact with the precision medicine data ecosystem using natural language, for both data management and insight generation. In response, conversational AI provides easily digestible outputs and insights without requiring users to navigate the complex underlying data structures.
- Prescriptive AI proactively serves up information around data anomalies and operational trends, then recommends potential actions to take based on this information.
- Navigational AI drives a re-envisioned user interface for precision medicine intelligence, guiding users to the specific data, insights, and modules of an application fit for their immediate needs.
Considerations for building a generative AI framework for precision medicine
As we build a generative AI framework out of an ensemble of conversational, prescriptive, and navigational LLMs, there are four main considerations to ensure that the resulting technology remains practical and usable:
- System and ecosystem interoperability via a scalable, modular approach
- Task and domain specificity, including specificity for user personas
- Compliance, including data privacy and security, without hampering innovation
- Balancing accuracy, speed, and cost efficiency
In this series of posts, we will explore the details of each of these considerations.
Follow QuartzBio for the rest of the story!
And if you’re interested in joining one of our in-person Biomarker Intelligence Summits for R&D organizations to share challenges and opportunities facing data-rich clinical programs, please get in touch.