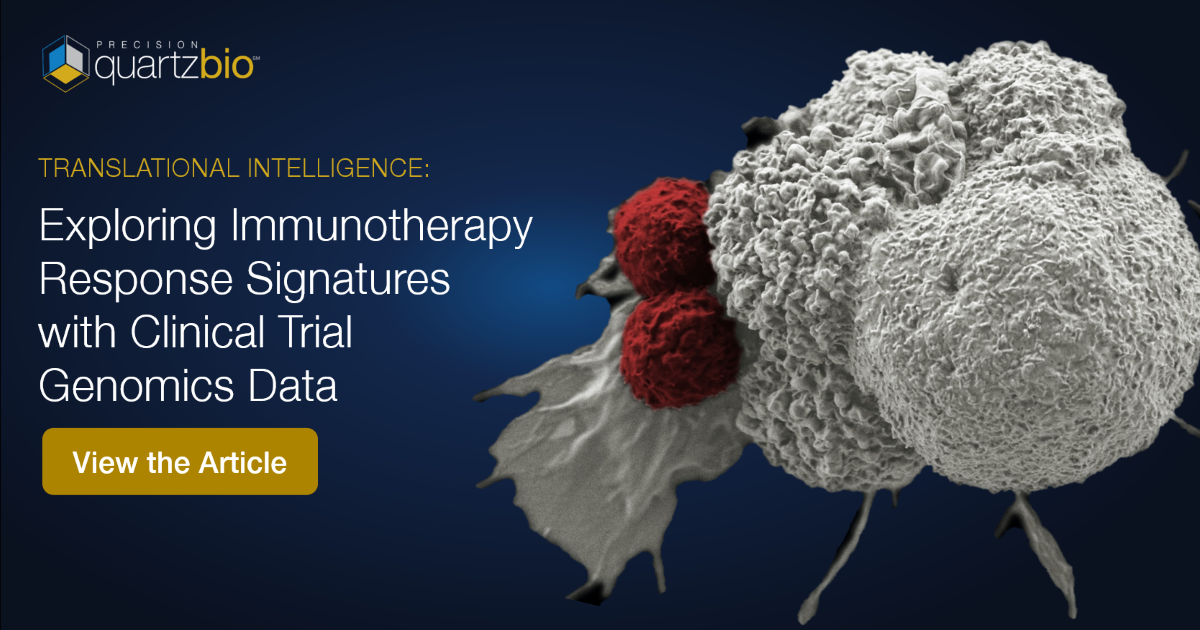
May 5, 2021 — As the data generated during a modern clinical trial has dramatically expanded, sponsors must continuously evaluate novel approaches to help bridge the gap between millions of data points and breakthrough scientific insights (a goal we describe as delivering Translational Intelligence). One such example is assessing signatures of “hot” and “cold” tumors.
Investigating Biomarker Signatures of “Hot” and “Cold” Tumors
For immuno-oncology programs targeting solid tumors, translational teams seek biomarker signatures that may be predictive of immunotherapy response. Tumors with significant tumor-infiltrating lymphocytes (TILs; “hot” tumors) have been observed to be more responsive to immunotherapy than tumors with lower levels of TILs (“cold” tumors).
Given the relative lack of definitive biomarkers of TIL infiltration status, it has been posited that both immune pathway activity and genomic mutation profile of tumors must be evaluated for better prediction of response to immunotherapy. Furthermore, interferon gamma (IFNγ) has been reported to increase TIL infiltration in “cold” tumors.
With interconnected genomic, transcriptomic, and clinical data as enabled by mapping, annotation and ontology engines, translational researchers and clinical scientists can assess the correlation between tumor mutational burden (TMB) in specific genes and expression of IFNγ signaling proteins, tumor volume, and, for example, a 193-gene immuno-oncology response signature curated from the literature (Figure 1).

Figure 1. Exploring the predictive value of genomic alterations in an immuno-oncology clinical trial. By correlating TMB at specific loci with tumor volume, IFNγ pathway gene expression, and curated IO response signature, the QuartzBio platform can be used to investigate predictive value of certain genomic alterations.
Genomic Biomarkers Used to Identify Patient Subgroups with Differential Clinical Response
Evaluating how likely a tumor is to respond to immunotherapy is a significant first step. However, we have also highlighted the value of connecting clinical, PK, and exploratory data. Once this data is harmonized, or “talking”, as we often say, it can be rapidly explored within the QuartzBio web application and via connectors to third party tools (e.g., GeneMANIA, Reactome, gnomAD). Figure 2 shows correlated mutation type/TMB/gene expression datasets being used to define patient subgroup(s) for further stratification and/or analysis. A click-and-drag TMB threshold was set (left) in order to assess the differential impact of the genomic alteration on overall survival (right).

Figure 2. Connect TMB at specific loci to clinical data to assess impact on survival, via interactive web application. The QuartzBio platform enables dynamic visualization of clinical data after correlation with genomic biomarkers. In this example, TMB at specific loci were correlated with overall survival. Setting a TMB threshold enabled the assessment of the impact of this genomic alteration on survival in the context of the clinical trial.
Genomic Insights Can Be Enriched with Knowledge Assets
Translational researchers can generate the most insights from clinical trial genomics and transcriptomics data by enriching this data with curated information. Curated biomarker signatures can be statistically correlated with experimental data to enable rapid scoring, as shown in Figure 1.
QuartzBio knowledge assets include
- Immuno-oncology Response Signature (193 genes)
- FOXP3 Signaling Signature (55 genes)
- IFNɣ Signaling Signature (1146 genes)
- CTLA4 Signaling Signature (13 genes)
What biological insights are your team seeking to extract from clinical trial data?
Join us for a webinar where Tobi Guennel at QuartzBio will present a case study on how teams are quickly identifying genes of interest and evaluating IO response signatures in their clinical trial genomic data.