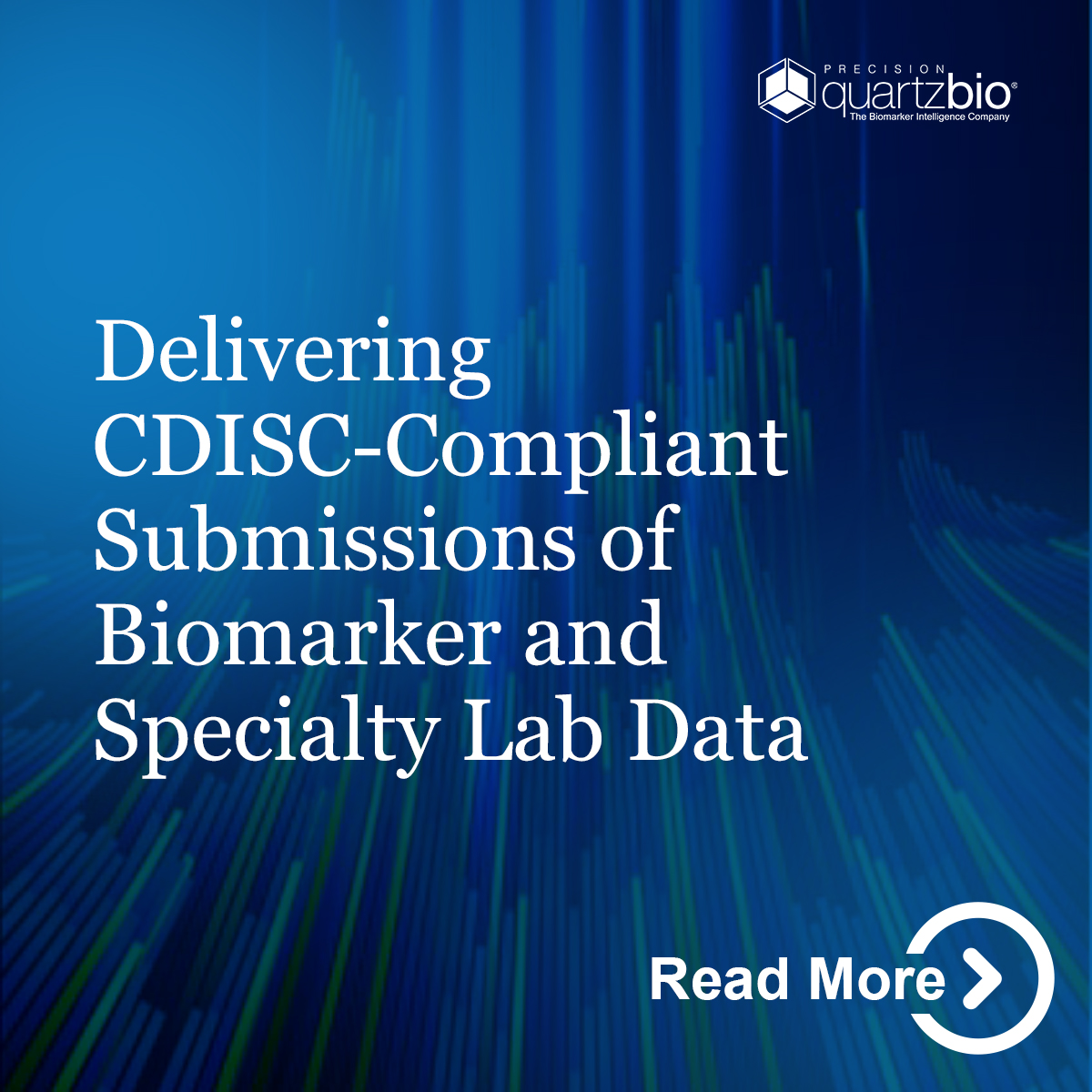
April 9, 2021 — With the rise of biomarkers used in clinical trials (e.g., prognostic, predictive, pharmacodynamic) and biomarker assay modalities (e.g. flow cytometry, multiplex protein detection, gene expression profiling), biomarker and specialty lab data are increasingly incorporated into FDA submissions. This data provides insights into key clinical objectives, including pharmacological effects, and drug safety and effectiveness.
Drug developers face operational challenges, however, in preparing complex, often unstructured, biomarker and specialty lab data in compliance with regulatory requirements.
Any study that began as of December 17, 2016 must use Clinical Data Interchange Standards Consortium (CDISC) standards, formats, and terminologies specified in the FDA Data Standards Catalog for New Drug Application (NDA), Abbreviated NDA (ANDA), and certain Biologic License Application (BLA) submissions.
The FDA specifies the use of CDISC standards, of which a subset is shown in Table 1:
CDISC Standard | Description |
---|---|
SDTM – Study Data Tabulation Model | SDTM files are standardized file formats required for the presentation of underlying raw data. Normally one SDTM file is prepared for each “domain” of data generated. |
ADaM – Analysis Data Model | ADaM is the dataset format required to capture the analysis of clinical data. Any transformation, baseline calculations, statistical transformations, or other data derivation that leads to statistical analysis must be captured in the ADaM file. |
SEND – Standard for Exchange of Nonclinical Data | SEND specifies how nonclinical data should be collected and presented in a consistent format. It is an implementation of SDTM for nonclinical studies. |
CDISC Controlled Terminology | List of acceptable, valid codes and values to be used with data items submitted to the FDA within CDISC-defined datasets. |
Table 1. CDISC standards govern data organization and analysis during the clinical research process.
Transforming biomarker data into CDISC-compliant datasets is typically a significant, multistep challenge – particularly in biomarker-rich therapeutic areas such as immuno-oncology or autoimmune disease. And in phase 1/phase 2 dose escalation and expansion studies, sponsors manage safety lab data (hematology and chemistry) and pharmacokinetics (PK) lab data in addition to clinical development biomarkers of pharmacodynamics and treatment response.
Clinical operations teams routinely prepare safety lab data and PK data using existing standards and technological solutions. On the other hand, managing clinical biomarker data is a relatively new discipline, and sponsors frequently encounter resource constraints as well as gaps in standards and technologies.
Technology Platforms Impact Efficient Delivery of Clinical Biomarker and Specialty Lab Data
How straightforward it is to transform clinical biomarker into CDISC-compliant datasets can depend on the technology platform(s) used for data processing as well as the domain structure of the SDTM.
Generating submission-ready data is standard practice when electronic data capture and electronic lab management tools are used to map data to a single, well-defined SDTM domain. This is the workflow commonly used by local labs at clinical sites generating hematology, chemistry, and other clinical data (Table 2).
By contrast, biomarker data generated by specialty labs and used in clinical development presents unique challenges given the diversity of data types, manual data processing (i.e., non-programmatic or absence of technology enablement), and breadth of SDTM domains.
Biomarker Data from Specialty Labs: Diverse Data Types, Manual Processing, Complex SDTM Mapping
Local Labs at Clinical Sites | Specialty Pharmacokinetics Group | Specialty Biomarker Labs | ||||||||||||||
---|---|---|---|---|---|---|---|---|---|---|---|---|---|---|---|---|
Example Data Types |
|
|
|
|||||||||||||
Data Processing Technology | Within EDC System
|
External to EDC System
|
External to EDC System
|
|||||||||||||
SDTM Workflow | Single Domain
|
Multiple Domains
|
Multiple Specialty Domains
|
|||||||||||||
SDTM Domains |
|
|
|
Table 2. Typical workflows for local labs, PK data, and specialty biomarker labs, showing the differences in data processing (eg, technology-driven vs manual) and SDTM mapping (eg, single, well-defined domain vs multiple, more complex domains).
3 Main Challenges Facing Clinical Biomarker Data
1. Complexity in biomarker assays. Raw biomarker data needs extensive processing before it can be mapped into SDTM. Data processing often requires deep understanding of the underlying biological assay.
For example, the reporter code count (RCC) files generated by Nanostring gene expression assays require sample level checks (RNA integrity, field of view ratios, and binding densities), background correction, and normalization.
2. Lack of structure in biomarker data. Biomarker data sources deliver disparate file formats (e.g., RCC, FCS, XLS) that exhibit inconsistent structure across assays and datasets. A lack of standards across labs further compounds the structural heterogeneity. Combined, the inconsistencies make it difficult to standardize downstream programming pipelines and maintaining traceability.
3. Incompatible submission timelines. Typically, sponsors must deliver submission-ready datasets at a specified time soon after database lock. In the case of safety lab data and PK data, the data preparation timeline is rapid and rigid. Increasingly, it is expected that specialty lab data will also be submitted according to these same timelines. But, because biomarker data are so much more complex and unstructured, it is counterproductive to add biomarker data handling to this timeline without resources and tools to transform these data efficiently in a quality manner.
Conclusion: Biomarker and Specialty Lab Data Domain Expertise Are Critical Considerations in Preparing Submissions
Historically, there has been a gap between management and more well-defined conversion of clinical (e.g. EDC) data to submission-ready formats. This intersection of specialty lab data expertise combined with CDISC/SDTM domain experience will continue to play an increasing role in modern drug development.
In our next post, we’ll highlight the value of technology-driven workflows in advancing biomarker data management and submission-ready biomarker statistics, ultimately enabling:
- Traceability from data generation through TLF
- Thorough analyses on tight timelines
- Shorter, more targeted, more efficient clinical trials
- Transition from exploratory programming environments to environments more seamlessly accepted by FDA (e.g., R to SAS environments)
In the meantime, contact us to learn more about our work.