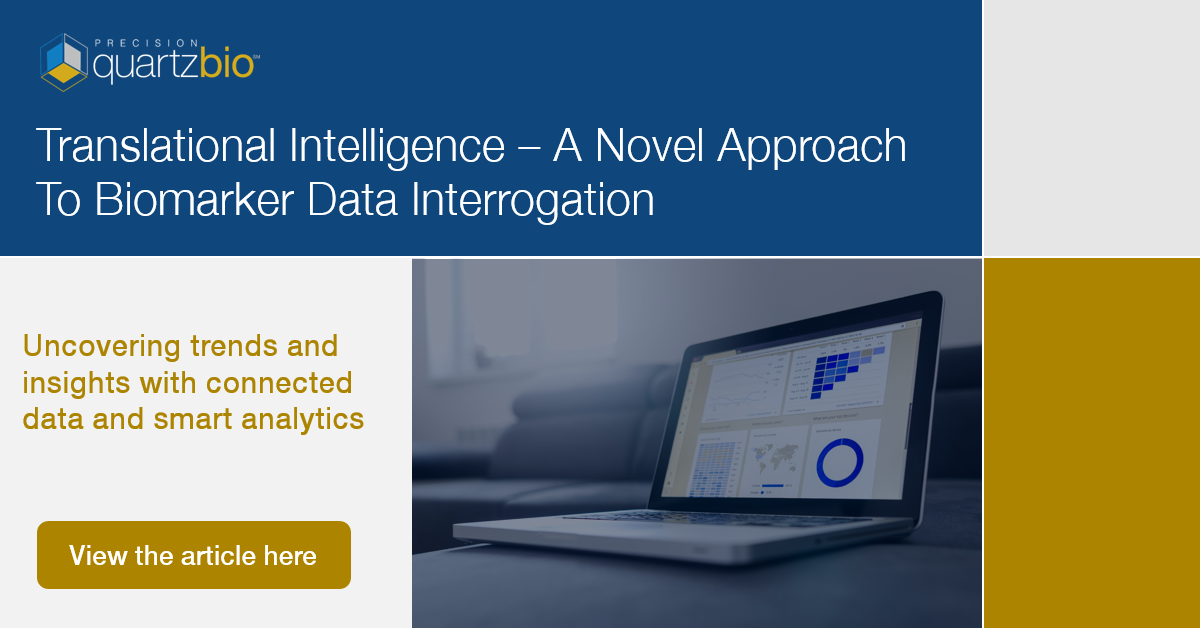
February 3, 2021 — In this brief, we explore Translational Intelligence – that is, navigating the vast biomarker data ecosystem to quickly uncover insights that would be missed by visual inspection alone, and which enable translational teams to focus on the right pathways, biological mechanisms, and / or patient populations.
In recent webinars and posts we’ve shared, our focus has been on:
- The operational challenges of biomarker-rich trials – challenges associated with all things samples (availability, consent, etc.) and assay data (data availability, projected data availability, etc.), and data and technology challenges.
- Opportunities to deploy technology-driven solutions to streamline management and integration of exploratory (e.g. flow cytometry, gene expressions), pharmacokinetics (PK), and clinical biomarker (e.g. IHC, targeted genomics) data
Biologically rich biomarker data generated in multiple labs
As we move further along the value chain (shown in Figure 1) from samples and results data, the next step is to extract translational insights that can advance drug development. Often, insights are derived by exploring correlative relationships between biomarkers known or assumed to be related to drug exposure, response, activity in the tumor microenvironment, etc.
Figure 1. Samples, Data, Insights as Core Value Chain
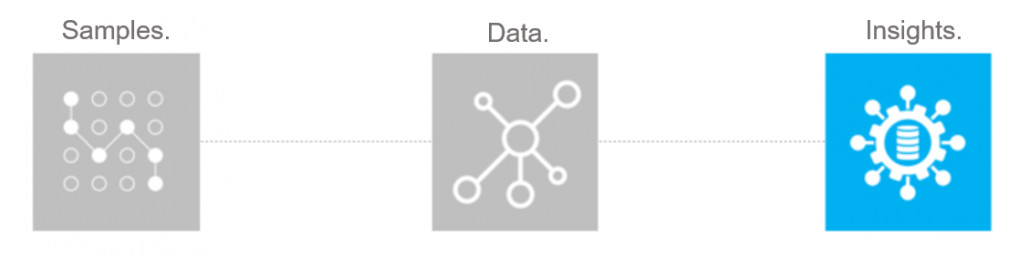
Consider a typical early phase (1 / 2) oncology, immunooncology (IO), or autoimmune clinical trial where robust biomarker data packages are generated throughout the life of the study. These data sets could include cytokine, gene expression profiling, flow cytometry, targeted capture, PK and clinical data. This results in tens of thousands of data points collected over time, across sites and labs with various source systems and technologies.
In a scenario in which the data from a study exists in an interrelated and connected database, we can unlock the ability to explore trends across assay/data types, trials, and programs. If we can sort through the noise and make our data talk, they can provide us unique insights related to program agnostic biomarker profiles of response, common dysregulated mechanisms, etc.
From Data to Insight with Translational Intelligence
While known biomarkers of interest are a critical starting point for analyses, a significant challenge is often determining what unidentified trends and relationships exist in the remaining tens of thousands of data points. There is a fundamental obstacle in analyzing exploratory data: it may not be clear which pathways, mechanisms or biomarker- defined patient populations to explore. These hypotheses are often constructed based on multiple biomarkers that span different biological variation (e.g., proteins and genes) that may not be elucidated in exploration of targeted biomarkers. This is where the notion of “Translational Intelligence” is useful.
Even within a completely connected data ecosystem, we need to rapidly synthesize high throughput (e.g. RNA-seq), high content (e.g. complex flow cytometry) data to surface complex relationships driven by biological signatures across assays (Figure 2). The goal is to uncover trends in study data that manual exploration alone could miss, and to help translational teams focus their efforts to maximize potential yield.
Figure 2. High-dimensional Biomarker Gene Expression Data
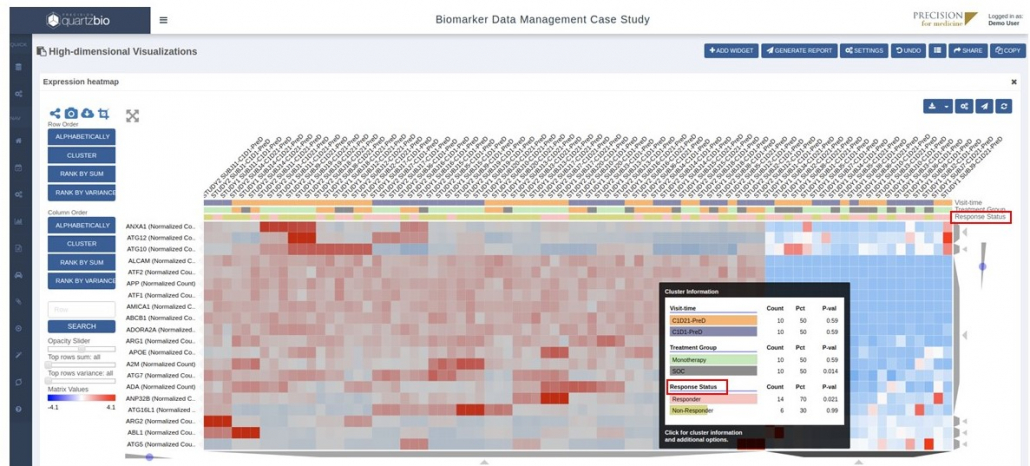
Figure 2. Synthesizing exploratory biomarker data with clinical data enables use of smart algorithms to flag trends for deeper exploration. This heatmap example shows a high-dimensional view of gene expression data, annotated with clinical metadata. Unsupervised clustering of this data allows for quick correlation between the metadata, such as response status (as outlined in red). These insights can inform pipeline decisions and prioritization.
Creating deeply connected data sets that “talk” to each other is the key step in enabling translational intelligence to maximize the utility and insight generation potential of every data point. This approach has the potential to uncover hypotheses (scientific questions) that might otherwise go unexplored, and to discover critical insights that might otherwise be missed.
In our next post, we’ll discuss more examples of what putting this into practice looks like, including through the utilization of smart algorithms.
Join our Translational Intelligence Webinar to see this technology in action!